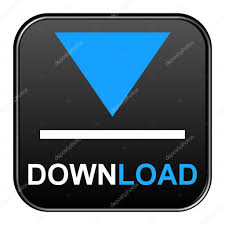

Ullamari Pesonen did not receive any fees related to this study.Ĭompeting interests: The Authors have read the journal’s policy and the authors of this manuscript have the following competing interests: Sammeli Liikkanen, Toni Sarapohja and Mikko Kuoppamäki are employees of Orion Corporation Orion Pharma, Finland. Institutional fees were paid for Valtteri Kaasinen, Eero Pekkonen, Filip Scheperjans and Mikko Kärppä. Tapani Keränen, Janne Sinkkonen, Joni Suorsa and Teppo Huttunen received consulting fees from Orion Corporation Orion Pharma, Finland. Sammeli Liikkanen, Toni Sarapohja and Mikko Kuoppamäki received salaries as employees of Orion Corporation Orion Pharma, Finland. Healthware Group participated in data collection. Orion Corporation participated in study design, data collection and analysis, decision to publish, and the preparation of the manuscript. This is an open access article distributed under the terms of the Creative Commons Attribution License, which permits unrestricted use, distribution, and reproduction in any medium, provided the original author and source are credited.ĭata Availability: Study report tables and listings: Wearable data set: 0MJFF dataset can be accessed through MJFF from: !Synapse:syn20681023/wiki/.įunding: This study was funded by Orion Corporation Orion Pharma, Finland, and Healthware Group, Italy.

Received: Accepted: MaPublished: April 7, 2023Ĭopyright: © 2023 Liikkanen et al. PLOS Digit Health 2(4):Įditor: Danilo Pani, University of Cagliari: Universita degli Studi Di Cagliari, ITALY (2023) Feasibility and patient acceptability of a commercially available wearable and a smart phone application in identification of motor states in parkinson’s disease. Finally, the usability of the data collection method is important to enable the collection of self-reported symptom data by PD patients.Ĭitation: Liikkanen S, Sinkkonen J, Suorsa J, Kaasinen V, Pekkonen E, Kärppä M, et al. The usability of the data collection method is important to enable the collection of self-reported symptom data by PD patients. Thus, a pre-detection of these states with a VAE from accelerometer data with good S/N ratio, and subsequent quantification of PD symptoms is a feasible strategy. A VAE model was able to detect the movement states. VAEs trained on either dataset produced embedding from which movement states in MJFFd could be predicted.

The analysis reveals differential symptom detectability across movement tasks, motivating the third part of the study. Discriminative power of linear models to separate symptoms from adjacent periods is weak, but aggregates show partial separability of patients vs. Similar spectral patterns also separate symptom periods from immediately adjacent non-symptomatic periods. Aggregated spectrograms of the collected accelerometer data show relative attenuation of low (<5Hz) frequencies in patients. Recording a symptom at the time of the event was assessed as very easy or easy by 70.1% (29/41) of subjects with PD. 88.9% (32/36) of PD patients, 80.0% (4/5) of DBS PD patients and 95.5% (21/22) of control subjects reported that using the wearable device was very easy or easy. A total of 7590 self-reported symptoms were recorded during the study. Variational autoencoders (VAE) were trained on both our study accelerometer data and on MJFFd to detect movement states (e.g., walking, standing). Accelerometer data from the Levodopa Response Study (MJFFd) were reanalyzed, with symptoms quantified with linear spectral models trained on expert evaluations present in the data. Subsequent analyses are based on continuous accelerometer data from the device. 42 PD patients and 23 control subjects were monitored with Garmin Vivosmart 4 wearable device and asked to fill a symptom and medication diary with a mobile application, at home, for about four weeks. The data collected from everyday life can be noisy and frequently contains artefacts, and novel detection methods and algorithms are therefore needed. The continuous, longitudinal, and automated detection of motor and especially non-motor symptoms with these devices is still a challenge that requires more research. Recently, also commercially available smartphones and wearable devices have been actively researched in the detection of PD symptoms.
#Reaktor library professional#
In the quantification of symptoms of Parkinson’s disease (PD), healthcare professional assessments, patient reported outcomes (PRO), and medical device grade wearables are currently used.
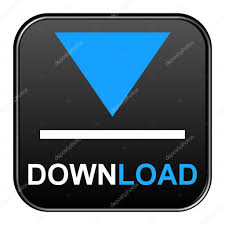